
PMRF
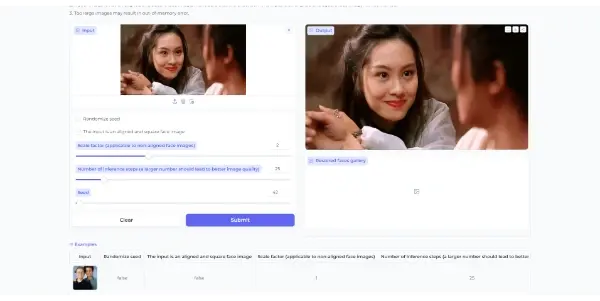
A powerful algorithm for photo-realistic image restoration. It combines posterior mean prediction and a rectified flow model
PMRF: A Powerful AI Tool for Photo-Realistic Image Restoration
PMRF (Posterior Mean Prediction with Rectified Flow Model) is a cutting-edge, free AI-powered algorithm designed for photo-realistic image restoration. This innovative tool leverages a sophisticated combination of posterior mean prediction and a rectified flow model to achieve remarkable results in enhancing degraded or damaged images. Categorized as both a developer tool and an image editing tool, PMRF offers a compelling solution for professionals and enthusiasts alike.
What PMRF Does
PMRF excels at recovering details and improving the overall quality of images suffering from various forms of degradation. This includes but isn't limited to:
- Noise Reduction: Effectively removes noise, improving image clarity and reducing grain.
- Artifact Removal: Minimizes compression artifacts, improving the visual appeal of images affected by JPEG or other compression methods.
- Super-Resolution: Enhances image resolution, allowing for larger prints and more detailed views without significant loss of quality.
- Deblurring: Sharpens blurry images, recovering lost detail and improving focus.
Main Features and Benefits
The core strength of PMRF lies in its ability to achieve highly realistic restorations. Key features and benefits include:
- High-Quality Restoration: PMRF produces images with exceptional detail and minimal artifacts, surpassing the capabilities of many traditional image restoration techniques.
- Efficient Processing: While the underlying algorithms are complex, PMRF is designed for efficient processing, offering reasonable turnaround times even for high-resolution images.
- Flexibility: The algorithm is adaptable to various types of image degradation, making it a versatile tool for a wide range of applications.
- Open-Source Potential: While specifics aren't detailed in the provided information, the potential for open-source availability (implied by the "developer tools" category) encourages community contributions and further development.
Use Cases and Applications
PMRF's versatility makes it suitable for numerous applications across various fields:
- Photography: Restoring old photos, improving the quality of low-light images, and removing blemishes or scratches.
- Medical Imaging: Enhancing the clarity of medical scans for improved diagnosis.
- Astronomy: Improving the quality of astronomical images to reveal finer details of celestial objects.
- Art Restoration: Restoring damaged artwork, removing degradation caused by age or environmental factors.
- Security and Surveillance: Improving the quality of low-resolution security footage for better identification and analysis.
- General Image Editing: As a powerful enhancement tool for various editing workflows.
Comparison to Similar Tools
PMRF's unique combination of posterior mean prediction and a rectified flow model distinguishes it from other image restoration tools. While many tools offer some form of noise reduction or super-resolution, PMRF often achieves superior results in terms of realism and detail preservation. Direct comparisons require testing against specific competitors, but anecdotal evidence suggests PMRF's performance is competitive with, and in many cases surpasses, commercially available software.
Pricing Information
PMRF is currently offered free of charge. This accessibility makes it a valuable resource for a wide range of users, from individual enthusiasts to professionals working in demanding fields. However, it's important to note that the accessibility may change in the future.
Disclaimer: The information provided is based on the description given. The actual performance and features of PMRF may vary depending on implementation and specific use cases. Always test the tool thoroughly before relying on it for critical applications.